Public access to Midjourney’s text-to-image AI fuels an explosion of creativity
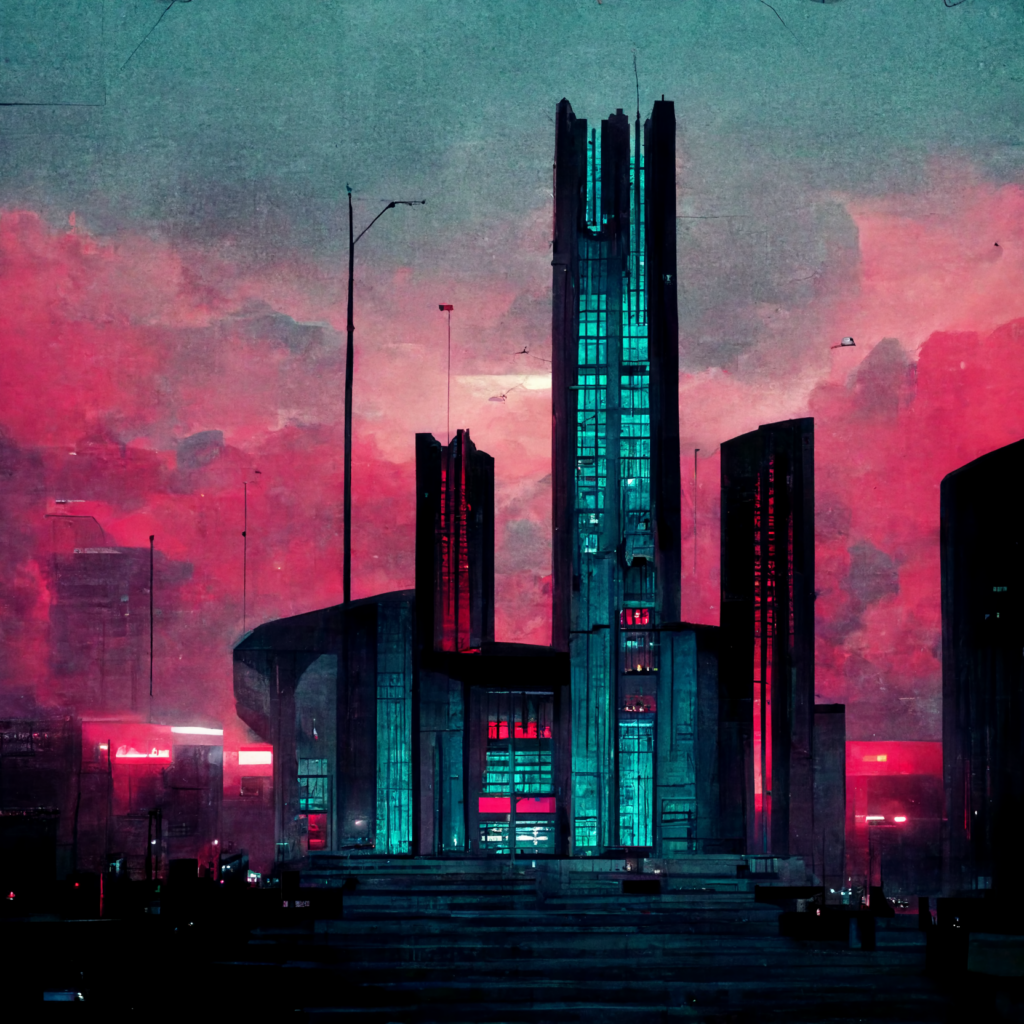
Every once in a while you come across something so amazing that it redefines how you see the world. Often that’s an idea that unlocks pathways to other ideas. On rare occasions it’s a person that’s lived a unique life, proving that something you thought impossible wasn’t actually. Sometimes it’s an experience that gives you a new perspective on your own life. All of these turning points come with a sense of the world becoming larger, of new opportunities unfolding in front of you. The temptation of curiosity then ignites the drive for exploration.
What’s currently happening in AI isn’t new to those who follow the space closely. Images created by OpenAI’s DALL-E 2 and others have been circulating for a while. That’s not the point here. A revolution doesn’t happen when someone shouts a brilliant new idea into a crowd. A revolution happens when people turn around to listen and then take matters into their own hands. That’s what’s currently happening with Midjourney’s AI system. It’s freely accessible in a quick and easy way through their Discord server and boy are people turning around.
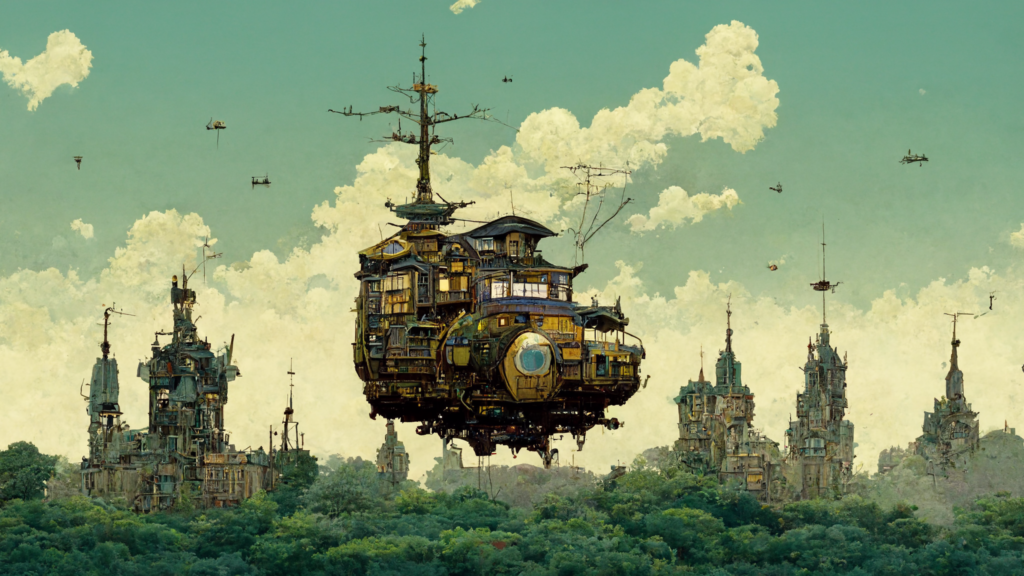
Yesterday evening at one point over 170.000 online users flooded the AI’s Discord bot with inputs, creating a never ending stream of fantastic, absurd, hilarious, inspiring and most of all new images. That last adjective is crucially important. Nobody has ever seen these images before. Aspects of it, sure, that’s how the AI works but the compositions themselves are novel creations. Dreams made reality with a few simple inputs. If you haven’t already done so – create a Discord account, join the server and watch this happening in real time. The technical details are secondary, it’s the social and economic phenomenon unfolding in real time that is fascinating. This is a moment that will be talked about for a long time.
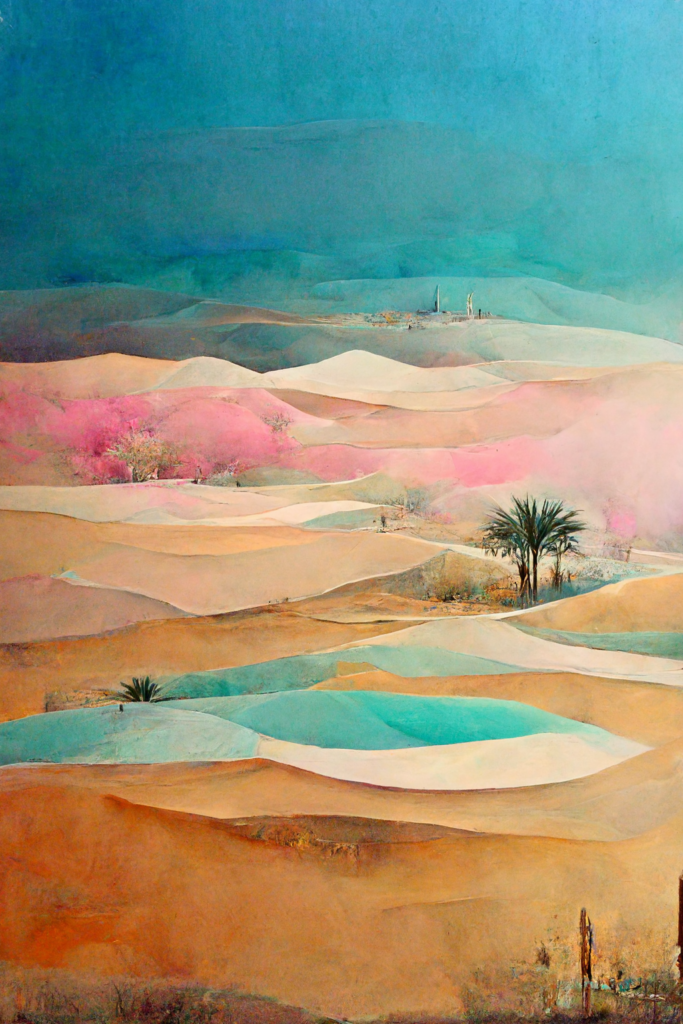
It’s not about seeing these images, it’s about creating them
Now you might look at the images I included in this post and think “that’s cool stuff, but I’ve seen cool stuff before – why is this dude getting so excited about a few images”. That was my first reaction as well. We’ve all been flooded by fantastic imagery and suffer daily from countless assaults on our visual cortex. And after all, this isn’t even video. The output of this AI, although fantastic, isn’t the main point. It’s having access to the creative process to get there that’s revolutionary. Let me explain.
The difference between watching someone else’s fantastic creations and creating your own is comparable to listening to someone talk about getting laid versus, well, actually getting laid (not saying that I’d ever place making cool images on the same level as the latter activity but you get the point). It’s this difference that makes people shrug when they see AI-generated images and the reason this didn’t get much traction outside of its niche before. It’s also the reason this is exploding right now. We’ve been handed the keys to the kingdom and the experience is quite hard to describe. As with the other thing, it’s better to just experience it.
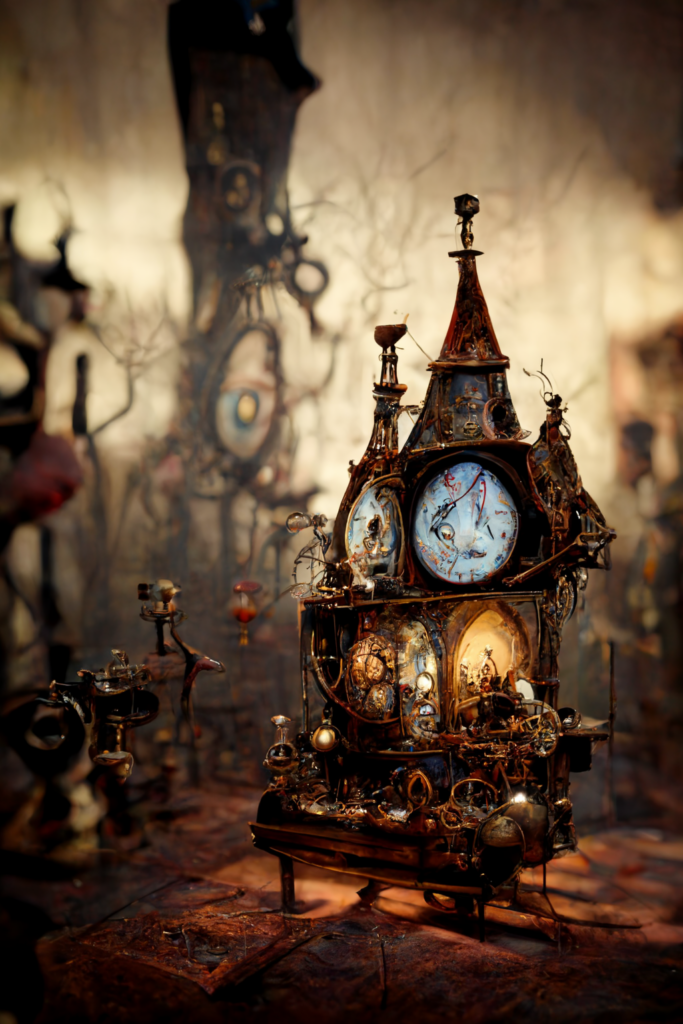
A breakthrough to a new frontier of human creativity
Okay, calm down there. We’re getting a bit ahead of ourselves here, aren’t we? Well, not really. Have you ever been to a museum, looked at a magnificent painting and thought to yourself “I have so many fantastic images in my head but no way to get them out of there”? After all, not many of us are born with the talent or the time to develop the skills it takes to translate our ideas into physical representations. That excuse doesn’t exist anymore, at least not in the same way. All you need to do now is to type out your dream and watch it getting painted. It’s a fantastic experience that changes the way you think about art forever. However, this is also where things get quite a bit more complicated.
The AI works by interpreting a string of text, which means the keys to the kingdom come in the form of having the ability to describe what you want the image to be composed of in a way that the AI interprets as you intend it to. For example, the previous image is the result of “American McGee’s Alice clockwork world, unreal engine, hyperrealist, –ar 12:19”. I found it amazing in many ways and decided to keep it but it’s nowhere near what I imagined. While it’s easy to get the AI to produce something, getting it to do exactly what you want is extremely difficult. It takes a new skill set. Enter the “AI whisperer”.
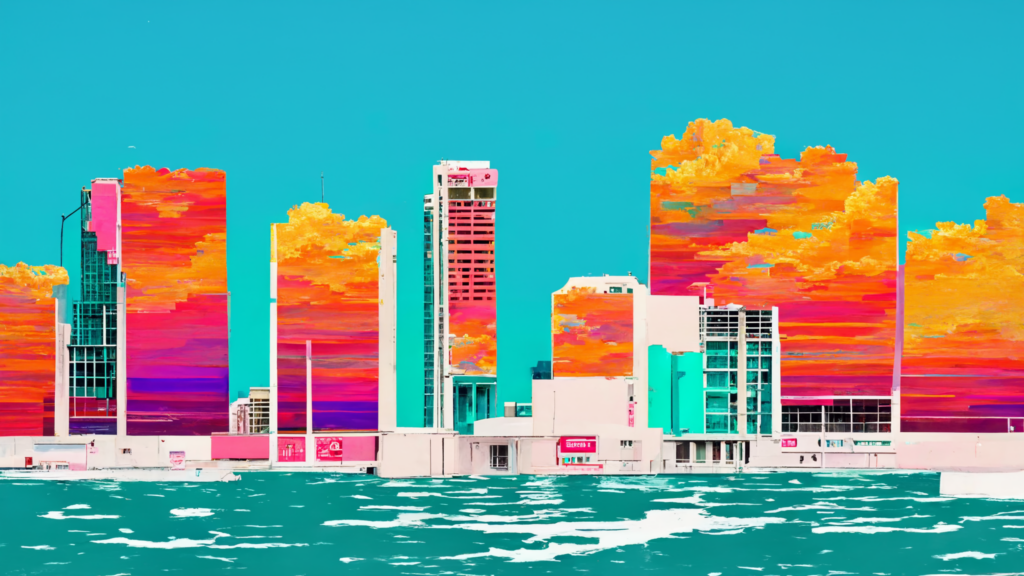
To create an image that mirrors your imagination you need to learn the AI’s way of understanding human language, at least for now. Alternatively you can spend hours trying to shape an image into what you want it to be by iterating through hundreds of incremental changes. That means you either need skill or time, the latter building the former. Learning from other people’s inputs is the glue that builds this new community, greatly speeds up the learning process and opens a pathway for anyone to turn themselves into an AI artist (hold your pitchforks, art people).
However, you quickly start to reach the limits of your creative vocabulary and a new frontier emerges. The good news is that your imagination isn’t limited by your ability to paint anymore. The bad news is that you’re now limited by your ability to translate your thoughts into text. Don’t get me wrong, that is a giant leap because it takes your body completely out of the equation. However, it also places you immediately in front of new challenges. If you want your image to look like the one by that great artist you saw once, well, you need to remember what the name of that person was and add it to your query (you can hear the collective sigh of the art people right there, can’t you?). If you want to create someone that’s truly new you’ll be busy for a while.
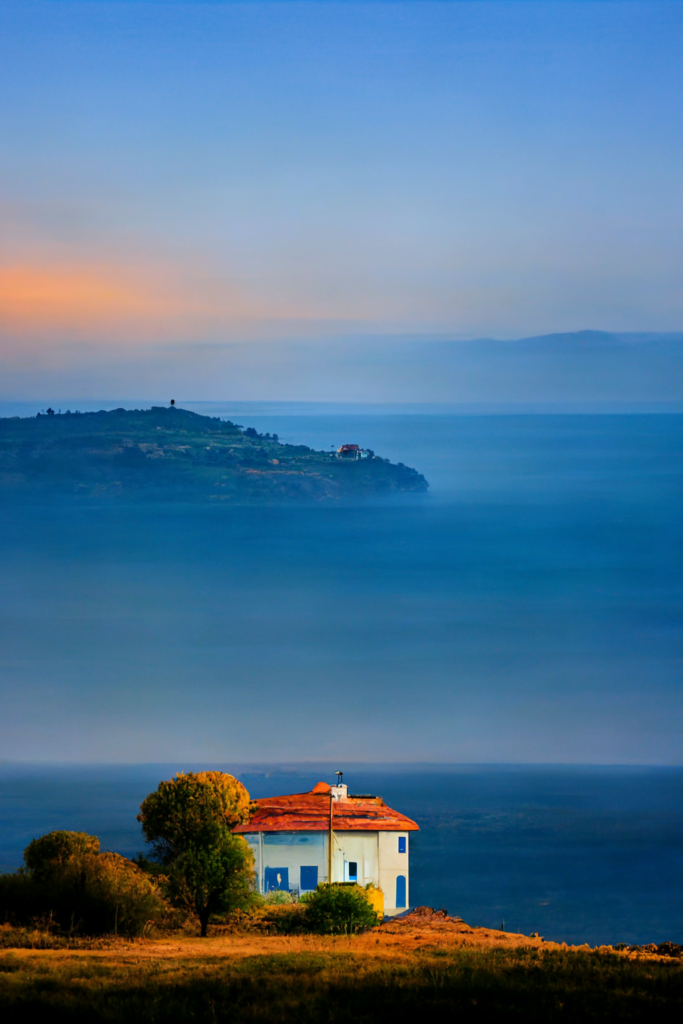
Free your mind (and your schedule)
All you are now limited by is your mind. And the time you can spend to provide feedback to the AI. And your knowledge of art, artists, styles, techniques, production methods and other useful details to improve the precision of your queries. And your English skills because inputs in other languages are even less precise. And your knowledge of technical commands like that “–ar 12:19” string I used above, which is used to tell the AI that I want an image with a 12:19 aspect ratio. Why exactly that? I have no idea, I just copied it from some dude who made a pretty image in portrait mode. You see where I am going with this. All of this is fun to explore and play around with but it’s also a new skill set, one that an artist is considerably better positioned to acquire in a reasonable amount of time.
Nevertheless, many entry barriers to the creation of beautiful imagery have just been removed. Just as the availability of free online education increased the chances of discovering the next Einstein and free sharing of audio and video increased the chances of finding the next Mozart, free public access to this technology increases the chances of discovering the next Van Gogh. Who knows, maybe future generations will one day study the revelatory incantations of FortniteGIGACHAD#1845.
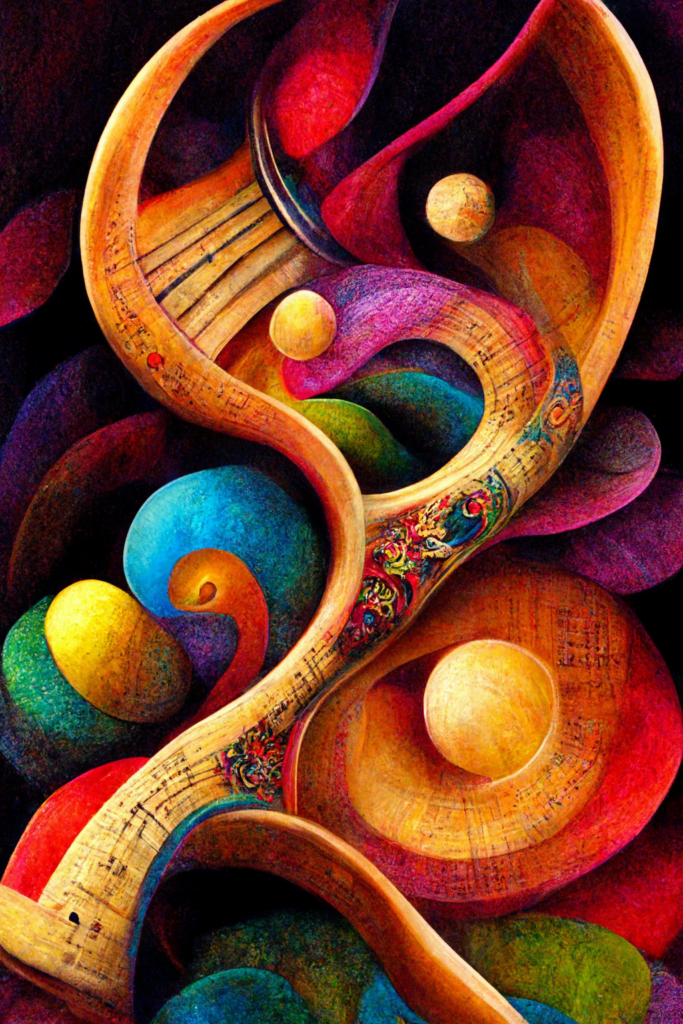
Enjoy the ride but fasten your seatbelts
Public access to creating digital art is a welcome side effect of advancements in AI. The bigger picture is that we’re all in for a wild ride. Midjourney’s CEO David Holz predicts that we might have an XBox with an AI to dream up entire games in less than ten years, video created in similar fashion in less than two. In comparison to that, building similar tools to write reports (or blog posts) seems like a fly on the windshield. If your job involves turning ideas, data and knowledge into text you might want to consider that the production process could change dramatically.
Holz is also mindful to point out that we don’t understand the technology’s humanistic implications. It is no accident that you see only positive images on Midjourney’s Discord server and I sincerely hope we never find out what we’re missing out on. I don’t think there has ever been a better practical example for the argument that any technology can be used for good and for bad. His point goes far beyond the concrete example I am picking but the conclusion is similar: we need to advance with caution.
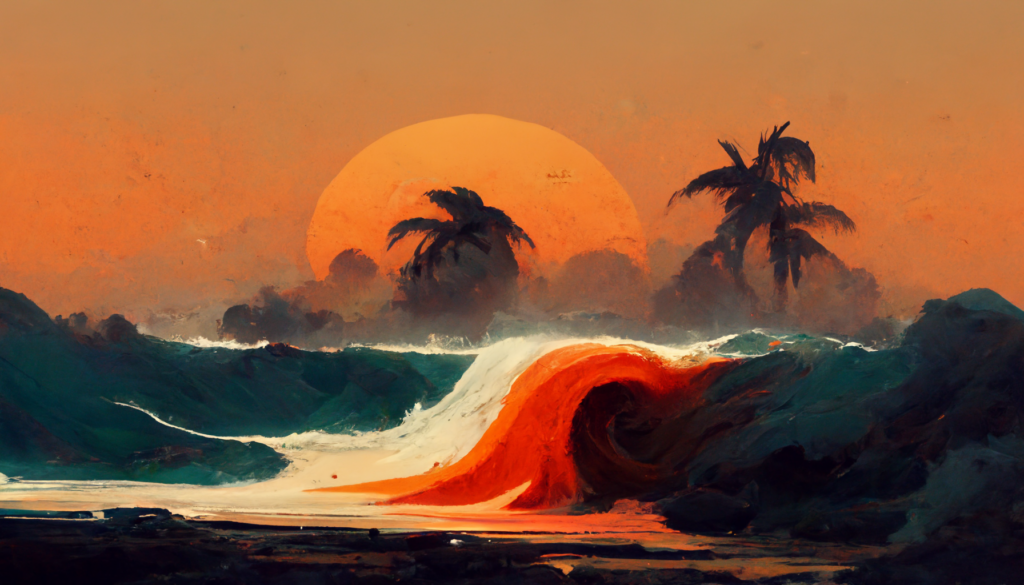
What remains in the end is pure excitement. Being able to translate the images of your dreams into reality feels like exploring a world you are building at the same time. When (not if) this tech masters another dimension and if (not when, unfortunately) access is granted to the public it will trigger an explosion of creativity and content not seen since Gutenberg’s printing press. Sounds absurd? Don’t forget that there is a historical reference point to support this claim. People were once granted inexpensive access to a version of this technology that could only place one pixelated block at a time. It became the most successful videogame in history.
Minecraft.